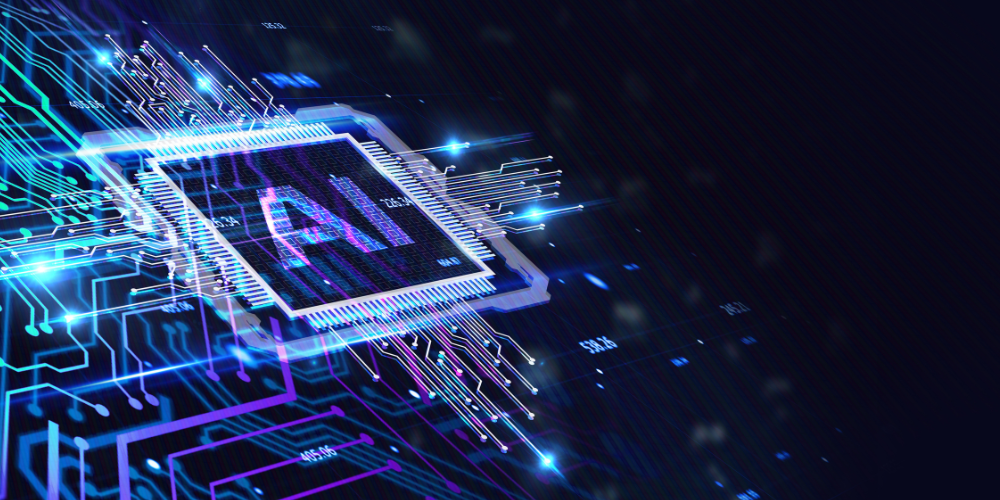
The Challenges Generative AI Poses for Data Centres
Artificial Intelligence (AI) has been described as a once-in-a-generation technology with the potential to transform huge swathes of our society, optimising operational efficiencies, driving productivity, supporting medical advances, and even solving some of the major challenges faced by the world today (such as climate change).
Many businesses, no doubt looking to gain a competitive edge, have already chosen to invest substantial amounts in in AI models and chatbots to automate functions such as answering customer enquiries, data analysis/modelling, and to take over redundant/repetitive tasks.
However, the rush to implement AI isn’t without risks. There are reports of considerable numbers of AI models/machine learning projects in large enterprises which have been expensive failures. Although the reasons haven’t been made public, there is clearly a need for companies to set clear goals and have a real understanding of the problems that AI can solve before committing development funds.
It's also apparent that while AI can carry out many tasks, and large language models (LLMs) provide astonishingly good content, the technology is currently fallible. AI models are trained using datasets and algorithms. They learn to make statistical predictions by finding patterns in the data and, in the case of LLMs, they are trained to predict the likelihood of a word or phrase within a given context; their understanding of language is mathematical. If, for example, the data is incomplete, or the model makes incorrect assumptions, it can offer false predictions known as hallucinating. That being the case, most commentators would agree that human oversight remains crucial particularly when it comes to automating critical business functions.
Successfully harnessing this transformative technology hinges on the capacity within data centres to train the models, and this is leading to new advances in data centre infrastructure.
How Data Centres Are Managing Demands of AI Training
Training AI models requires vast quantities of data as well as immense processing power. Generative AI (Gen AI) models, for example, use large neural networks consisting of hundreds of billions of parameters, and they rely heavily on Graphics Processing Units (GPUs) for training. GPUs are notorious for their high power demands - hence why industry projections suggest that data centre energy consumption is poised to skyrocket in the coming years, driven primarily by the growth of AI and AI training.
Data centre operators are also having to manage the sharp rise in thermal loading within racks, caused by all this processing power. It’s an issue which is exacerbated by the fact that electrical components are shrinking in size, and rack densities are rising, trapping more heat inside. This heat must be removed or it will damage the equipment’s delicate internal electronic components.
For higher density facilities where rack loads are reaching in excess of 40-50kW, liquid cooling packages can be installed within the rack suite to deliver cooling directly where it is needed. They are very energy efficient and so can help reduce the data centre’s carbon footprint. Furthermore, they are easily scalable to accommodate any future expansion.
However, once heat densities escalate further into regions exceeding 200kW air cooling is no longer a feasible option. Instead, two other liquid cooling methods are seeing large interest in advanced installations.
One method is immersion cooling, a technique where servers are submerged in tanks containing a dielectric fluid. This is a more efficient solution, but it comes with its own set of complexities, such as concerns over whether it invalidates equipment warranties as well as a need for robust structural floors to support the additional weight of the tanks used.
The second method is on-chip cooling, where liquid coolant is pumped through pipes directly to heatsinks on the chips where it absorbs and removes excess heat. Its many benefits for data centres include reducing energy consumption, increasing processing capacities, and improved uptime. Plus, it doesn’t take up as much space as immersion cooling
For data centres with racks that have a lower power requirement, air cooling (in-aisle) may be sufficient but, for the most part, liquid cooling delivers the greatest benefits.
Once trained, AI models can be integrated into operational applications by companies. Here, the focus shifts towards a requirement for real-time data processing by the technology; a process that requires low latency. In practice, this means placing the IT equipment close to the source of data generation within the workplace, in areas such as on factory floors.
This close proximity to the data source will minimise latency but can bring new issues, not least the problems caused by placing delicate electronic components in less-than-optimal operating environments (e.g. in hot, dusty, humid, industrial settings). These operational demands, coupled with the potential stresses on the equipment, have led to a steep rise in the number of so-called edge data centres deployed within companies.
Edge data centres typically use pre-configured modules that include built-in cooling, power supply, and IT racks. They are quick and easy to install and give businesses great opportunities for further scalability.
Conclusion
While we are expecting AI to help businesses unlock a new era of innovation and efficiency, successful deployment of AI models hinges on a balanced approach that acknowledges its limitations and ensures continued human oversight. But we must not lose sight of the demands that training AI models is placing on data centres, particularly in terms of energy and cooling.
If we are to realise the full potential of this technology, then data centre operators need to take full advantage of the latest generation in data centre infrastructure, otherwise the speed of progress and future developments could stall.